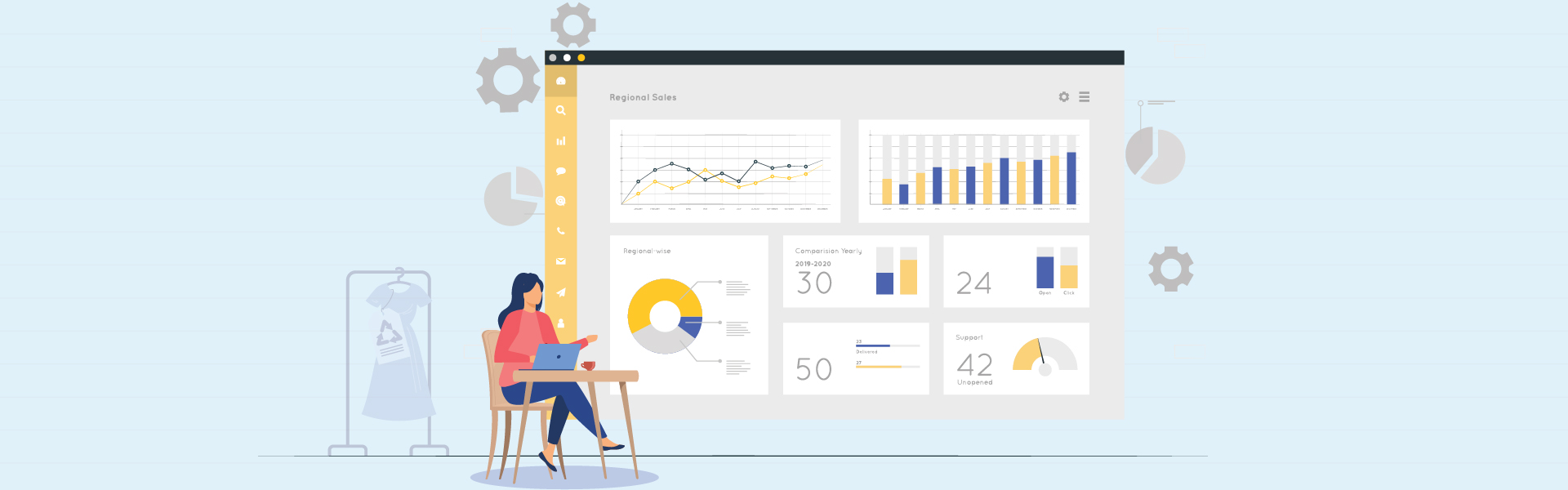
Client Introduction
The client is a pioneering fashion brand renowned for its sustainable and innovative apparel. They use cutting-edge technology to create fabrics that repel liquids, stains, and odours, offering functional and stylish clothing that reduces environmental impact. With collections available in major cities and select international markets, they provide high-quality, eco-friendly fashion to discerning consumers.